GeoWeaver: Improving Workflows for AI and Machine Learning
February 17, 2020
When it comes to artificial intelligence (AI) and machine learning (ML), researchers are often stuck managing workflows on their own. The variety and complexity of ML models and the tremendous number of disparate tools and platforms make solo management a challenge, especially when big Earth data is involved. GeoWeaver is the open source workflow management solution that many AI practitioners urgently need.
GeoWeaver is a web-based and multi-user workflow management system (WfMS). Funded by NASA’s Advancing Collaborative Connections for Earth System Science (ACESS) program, a new project will expand GeoWeaver into an effective platform to improve AI-based workflows in their ability to share, replicate, and reuse.
This collaboration began with Dr. Ziheng Sun’s (George Mason University) small incubator project funded by the Earth Science Information Partners (ESIP). Sun’s progress and vision for future work caught the attention of Dr. Xiaogang “Marshall†Ma (University of Idaho). They found common interests in scaling up the power of cloud-based workflow platforms, automating provenance documentation for open science, and boosting the development of explainable AI. Together with Dr. Annie Burgess (ESIP) and Dr. Daniel Tong (George Mason University), they received a $900,000 NASA ACCESS grant and will collaborate on the GeoWeaver project for the next three years.
GeoWeaver allows users to manage data, servers, scripts, and notebooks all in one place. Ma says, “It will bring great benefits to the AI community to build, run, share, track, modify, reproduce, and reuse their AI workflows in either a single-machine or distributed environment.†In this way, GeoWeaver could become a fundamental tool for research topics that use geospatial data, like forestry, agriculture, ecosystem, and geology.
The proposed AI workflow management framework.
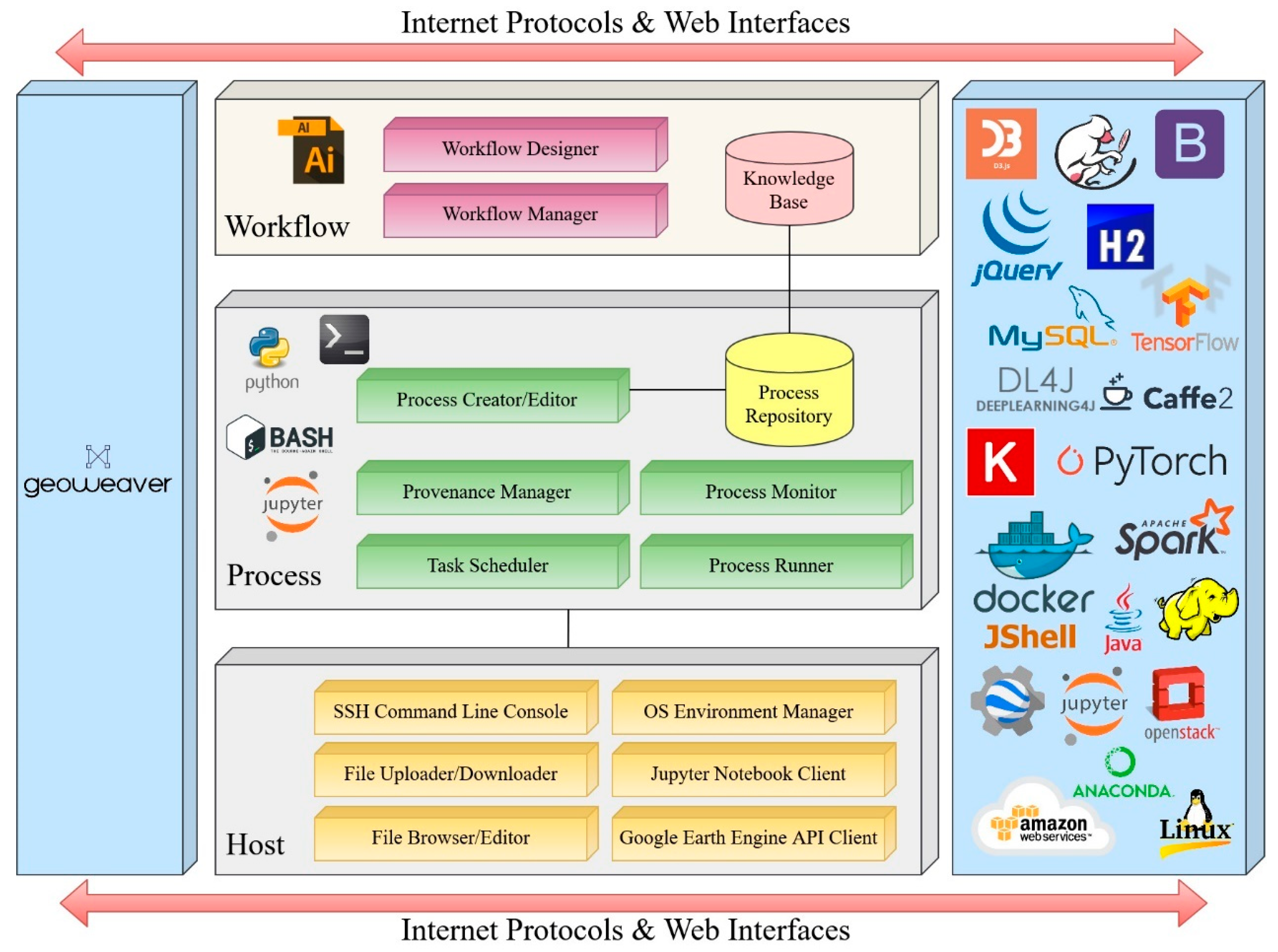
GeoWeaver is scheduled to be used for NASA’s Earth Observing System Data and Information System (EOSDIS). It also has broad potential applications in Ma’s NSF EPSCoR Track 2 project to leverage big data for studying spatiotemporal patterns in tick-borne disease. Consequently, the Track 2 project will provide use cases to test and improve the usability of GeoWeaver.
Ma’s key work in this project will involve provenance documentation—building ontologies based on community standards to formulate GeoWeaver code history into a machine-readable and interoperable format. This will enable the connection of variables, model outputs, research activities, and scientists with their supporting datasets and data analysis methods.
“GeoWeaver serves as a user-friendly entry point to incorporate large datasets, high performance computation, workflow platforms, and cloud environment, so it will cost less time for new users to get familiar with and leverage the infrastructure,†says Ma. To improve usability even further, the team will provide detailed tutorials on the website and organize seminars for interested users.
In its finished state, GeoWeaver will feature hybrid workflows, full access of remote files, hidden data flows, separation between code and code execution, and process-oriented provenance to allow reproducibility and open science.
An initial prototype of GeoWeaver has already been released through the ESIP incubator project. Ma says, “With the new support from the NASA ACCESS grant, our team will further develop GeoWeaver into a reliable production-level software in the next three years.†They plan to build a central catalog for public workflows, test workflow transfer between GeoWeaver and other facilities, and create a list of best practices using data from NASA and other sources.
Article by Katy Riendeau
IBEST Design & Marketing Coordinator